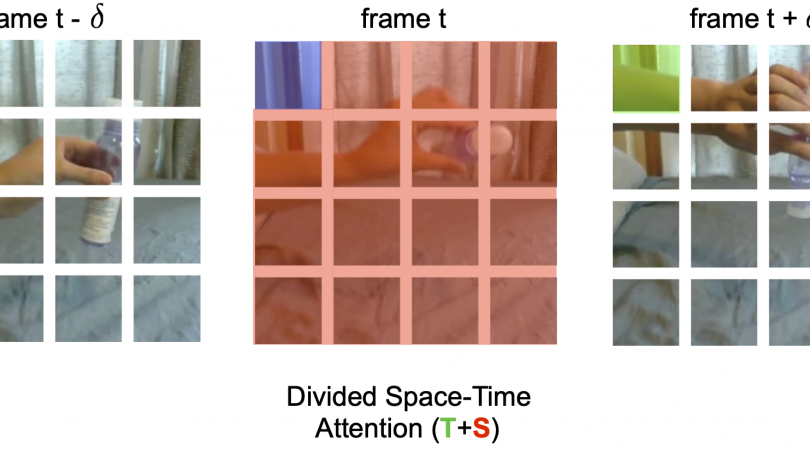
Menu
- Undergraduate
- Graduate
- Research
- News & Events
- People
- Inclusivity
- Jobs
Back to Top Nav
Back to Top Nav
Back to Top Nav
Professor Lorenzo Torresani, together with collaborators at Facebook, has developed a new deep-learning architecture for automatic video understanding that is more accurate and more efficient than the state-of-the-art. It follows a radically novel design inspired by deep networks used in Natural Language Processing (NLP). "Machine learning researchers have shown that it is possible to train neural networks that better comprehend natural language by means of a mechanism known as self-attention" says Torresani. "Self-attention models compare each word in a sentence with all the neighboring words. This operation is effective at disambiguating the meaning of words that require context from the entire sentence in order to be fully interpreted. Examples include pronouns or words that have multiple meanings."
Professor Torresani's architecture, named "TimeSformer," extends the self-attention operation from NLP to the video setting. "Our model views the input video as a space-time sequence of image patches, analogous to a sequence of words in a document. It then compares each individual patch to all the other image patches in the video. These pairwise comparisons allow the network to capture long-range space-time dependencies over the entire video and to recognize complex activities performed by subjects in the sequence."
TimeSformer is the first video architecture purely based on self-attention. Compared to existing models for video understanding, TimeSformer achieves higher action classification accuracy, is faster to train and can process video clips that are much longer (over a minute long as opposed to only a few seconds).
Facebook has posted a blog article describing this research.